EPRI Uses Deep Learning for Eel Conservation in the Saint Lawrence River
Young salmon swim down rivers through hydroelectric dams to the ocean. As adults, they swim upstream to spawn, using fish ladders to bypass the dams. American eels also navigate dams when it’s time to reproduce but do so in reverse. After maturing upstream, they swim downstream to ocean breeding grounds.
Unlike salmon, eels are fully grown—up to three feet long—when they pass through dams. This makes them particularly vulnerable to turbine blades. Less than 80% of eels survive passage through a single dam, and far fewer survive passage through multiple dams. Since 2014, EPRI’s Eel Passage Research Center has been investigating how to guide eels around the two hydropower facilities along the Saint Lawrence River, which connects the Great Lakes with the Atlantic Ocean. Promising methods include using light or sound to direct eels away from the turbines.
An important part of this research is tracking eel movements. For migrating salmon, researchers use acoustic tags to track movement and can observe them through windows at the top of fish ladders. Eel tracking methods are less advanced.
“To develop technologies to prevent eels from entering turbine intakes, we first need to observe and understand their downstream movement,” said EPRI Principal Technical Leader Paul Jacobson.
This is not easy in the Saint Lawrence River. The river is wide, deep, and fast-moving, and eels migrate primarily at night. The Eel Passage Research Center examined the use of sonar to monitor eels as they migrate downriver. Sonar sends out sound waves that bounce off underwater objects (such as eels) and return to a detector, generating images of the objects.
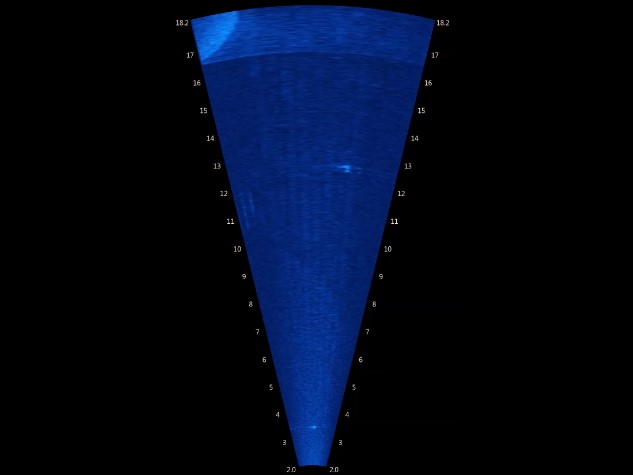
Along the upstream side of the Iroquois Dam on the Saint Lawrence River, Jacobson’s team set up sonar systems with a range of 30 meters. The devices scanned for eels swimming normally through the dam as well as for eels tethered to floats (to produce images certain to contain eels). Eels, sticks, vegetation, and other fish species appear in the sonar images as bright areas. Human analysts examined the sonar images to distinguish eels from other objects. Because eels are relatively rare, false positives can easily skew eel counts.
“Humans are very good at image analysis and can easily classify images of familiar objects,” said Jacobson. “For example, most people have no trouble identifying brands and models of cars.”
The sonar analysts correctly identified 88% of the eels in the images and never mistakenly identified other objects as eels. However, the process is time-intensive and requires analysts familiar with eel sonar images.
With funding from the U.S. Department of Energy, EPRI and Pacific Northwest National Laboratory are investigating the use of deep learning algorithms known as convolutional neural networks to analyze the large sonar data sets. Researchers trained the algorithms using images of eels and other objects from the St. Lawrence River and the laboratory. They tested the algorithms using similar data not used during training and assessed the accuracy. The algorithms achieved accuracy equal to or better than that of the human analysts.
The technology has potential to quickly provide the consistent, reliable data that dam operators may need to satisfy licensing requirements. Ultimately, data could be processed in real time, improving facility operations and making data storage unnecessary.
Jacobson sees an opportunity to improve the algorithms’ performance. With the current approach, the algorithms classify consecutive images of the same eel independently, ignoring the swimming motion revealed by image sequences. By including motion analysis, Jacobson expects the algorithms to yield accuracy far superior to that of human analysts. EPRI and Pacific Northwest National Laboratory plan to examine this in a follow-up study.
Jacobson says that automated eel monitoring tools can help researchers at the Eel Passage Research Center develop technologies to steer eels away from turbines. The deep learning software is open-source and available for others to use.
“We hope the technology will be widely adopted,” said Jacobson. “It can be useful for power companies facing similar eel management challenges in Europe, New Zealand, and Australia. It can also be adapted to track other kinds of fish such as lampreys and sturgeon.”
Key EPRI Technical Experts:
Paul Jacobson
For more information, contact techexpert@eprijournal.com.
Additional Resources:
Artwork by Ariel Davis