EPRI has embarked on a four-year effort to build a comprehensive dictionary of electric power industry terms aimed at advancing the industry’s adoption of artificial intelligence (AI) technologies.
As part of planning, operation, and training activities, electric utility staff often need to review many long documents, find data in the documents for subsequent analyses, and synthesize the information into actionable results. These activities are time-intensive and often tedious. In nuclear plants, for instance, workers spend a considerable amount of time combing through plant maintenance logs, regulatory reports, inspection reports, and operating experience reports.
“Many tasks in nuclear plant operations require analysts to search for information in numerous technical documents that can be hundreds of pages long,” said Carola Gregorich, an EPRI researcher investigating the use of AI in the nuclear power industry. “These tasks can take hours or days, and the analysts may not even find what they are looking for.”
Natural language processing (NLP)—a type of AI that reads, understands, and analyzes human language—offers the potential to automate these activities and complete them much more quickly. While NLP has been researched for decades, only recently has it advanced sufficiently for commercial applications, such as smart speakers, word suggestions for texts on smartphones, Web searches, and chatbots that can answer questions from customers.
Because language is inherently ambiguous and word meanings vary by context, NLP algorithms need a reference or dictionary to understand and analyze text. Dictionaries for NLP applications typically do not contain definitions; rather, they include words and phrases, their variations, and their associations with other words.
Open-source NLP dictionaries such as Wordnet have been successfully used for analysis of commonly used language, but they don’t understand the power industry’s unique technical terms, phrases, acronyms, and abbreviations. For example, the nuclear industry term “drain cooler relief valve” is a compound noun that refers to a specific type of relief valve found in nuclear plants. Generic NLP dictionaries would divide the phrase into separate words—drain, cooler, relief, and valve—and therefore not understand its intended meaning.
Recognizing these limitations, EPRI researchers have started to develop a power industry–specific dictionary for NLP applications. When completed, it would be available for use by utility staff.
“The vision is to create a large, comprehensive power industry dictionary comprised of numerous, separate sub-dictionaries, each focused on specific technical areas,” said Gregorich. “The terms in the sub-dictionaries would be transferrable to other sub-dictionaries. As more sub-dictionaries are created, they would be merged with the overall dictionary—similar to building with LEGO bricks. It would be a living dictionary, continually expanded and refined.”
EPRI has started the first sub-dictionary, which is focused on groundwater protection at nuclear plants.
“Protecting groundwater from spills and leaks is a high priority for nuclear plant operators,” said Gregorich. “We want this dictionary to support NLP algorithms that can analyze industry operating experience to yield new insights on how to reduce the risk of leaks and spills—or prevent them altogether.”
To build the dictionary, a collaborative team of groundwater experts and data scientists used various NLP algorithms to process a broad set of groundwater-related documents from EPRI, the Institute of Nuclear Power Operations, and the U.S. Nuclear Regulatory Commission. The algorithms identified key terms and phrases, their relationships with other words, and their variations (for example, “groundwater,” “g water,” “gnd water,” and “GW” all have the same meaning).
The current dictionary, which contains about 900 words and phrases, can be used by NLP tools to understand the language in similar documents and process them for answers to groundwater research questions much more comprehensively and much faster than any human. The effort demonstrated a repeatable process for building dictionaries specific to a technical area. Over the next four years, the team plans to apply the process to create dictionaries for several other technical areas, including:
- Nuclear plant maintenance: NLP algorithms can evaluate large amounts of maintenance-related documents (such as maintenance logs and work orders) for insights on the root causes of component failures, enabling plants to improve maintenance strategies.
- Corrective Action Program: Each year, a nuclear plant generates an average of 10,000 Corrective Action Reports, which evaluate the safety and reliability implications of problems, observations, near-misses, and other plant incidents. Activities associated with these reports are time-intensive: Each day, several managers may each spend hours reviewing the reports, assessing the severity of the incidents, and setting priorities for addressing them. With NLP, this process can potentially be streamlined, reducing staff time and effort by 80%.
- Performance of grid assets: NLP techniques have shown early promise in analysis of transmission and distribution asset performance. In particular, EPRI has found them useful in analyzing descriptive maintenance and outage records to yield actionable insights, such as the cause of equipment outages and the most common maintenance actions for different equipment families, makes, and models. The next step is to make the techniques more powerful by applying them to larger datasets compiled by pooling equipment maintenance records from across the industry.
- Automated component tagging: Utilities’ monitoring and diagnostics centers gather data on power plant operations across their fleets and analyze that data for insights on the performance of components and systems—and for early signs of failures. These analyses are challenged by the fact that different plants and manufacturers use different conventions and standards to name or “tag” components. EPRI is developing an NLP tool that can search through all the tags in incoming data to create standard names for each component, supporting more efficient, robust analyses of fleet performance.
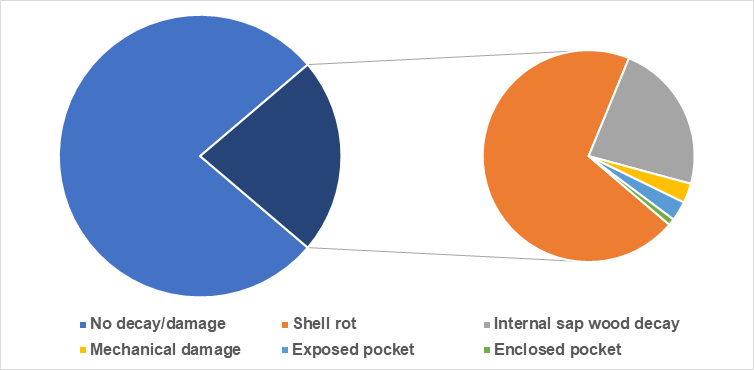
“EPRI is in a unique position to create dictionaries and use NLP methods for different applications in the power industry,” said Jeremy Renshaw, manager of EPRI’s AI Initiative. “We have access to more than 25 million industry-related reports, and each one represents a dataset that we can use to refine the dictionaries and train NLP algorithms. Our ongoing collaboration with utilities enables us to get even more data.”
Key EPRI Technical Experts:
Carola Gregorich, Chris Wiegand, Jeremy Renshaw, Bhavin Desai, Lea Boche, Yashwant Jankay
For more information, contact techexpert@eprijournal.com.
Additional Resources:
Artwork by James Provost