EPRI Looks at Machine Learning and Synchrophasor Data to Find Problems on the Grid
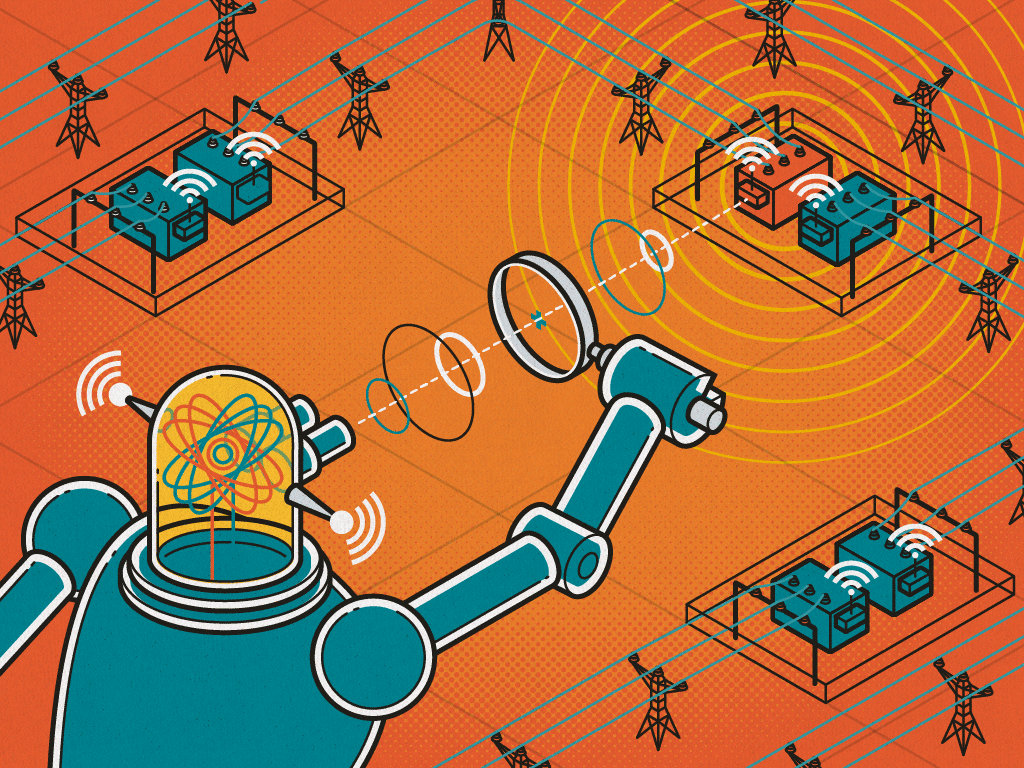
In the future, artificial intelligence could help grid operators avoid outages. In a preliminary EPRI study, machine learning techniques showed promise in their ability to accurately identify events on the power grid by analyzing massive amounts of data.
Over the past decade, utilities have deployed thousands of phasor measurement units in grids across the United States. These collect synchronized voltage and current measurements (known as synchrophasors) 10–60 times per second. For grid operators, these data can potentially provide better awareness of real-time conditions, help integrate renewable energy, and help detect and identify problems before they lead to outages. Machine learning seems poised to help utilities make sense of this and other vast data sets.
To analyze the synchrophasor data, EPRI investigated the applicability of supervised and unsupervised machine learning techniques and proposed frameworks that combine them. Supervised learning tends to be more accurate but requires data labeled with grid events. When such data were not available, unsupervised learning was used. Preliminary experiments used simulated data.
EPRI plans to apply the technique to real synchrophasor data and develop software to perform the analysis, with the first version expected by the end of 2019.
Key EPRI Technical Experts:
Evangelos Farantatos
For more information, contact techexpert@eprijournal.com.
Additional Resources:
- Data Mining Techniques and Tools for Synchrophasor Data (a public report from the North American SynchroPhasor Initiative)
Artwork by James Provost